However, the risk of bias playing a major role in determining the outcome of loan applications using AI has never been greater.
By Michael Akuchie
Lendsqr, a Nigerian fintech company focused on lending software, recently disclosed that it is building an AI-driven loan screening system that will automate the loan application process. Typically, a person seeking a loan must first submit an application and, after several rounds of background checks, may either be approved or denied. This process relies heavily on human deduction—a skill traditionally exercised by loan officers.
Lendsqr’s proposed project offers a more cost-effective approach to screening loan applications. Rather than following the traditional format of filling out paperwork and awaiting an interview with a loan officer, applicants will instead interact with the AI system via audio or video chat.
Structured like a typical conversation, the applicant will provide information about their employment and how they intend to repay the loan. Additionally, the system will analyse the applicant’s bill payment history as part of its efforts to make a holistic decision.
If successful, this project could mark a significant shift in how loan applications are handled in Nigeria and across Africa. However, the risk of bias influencing AI-driven decisions has never been higher. In recent years, companies across various industries—such as transportation, manufacturing, and healthcare—have adopted AI to boost operational efficiency while reducing costs.
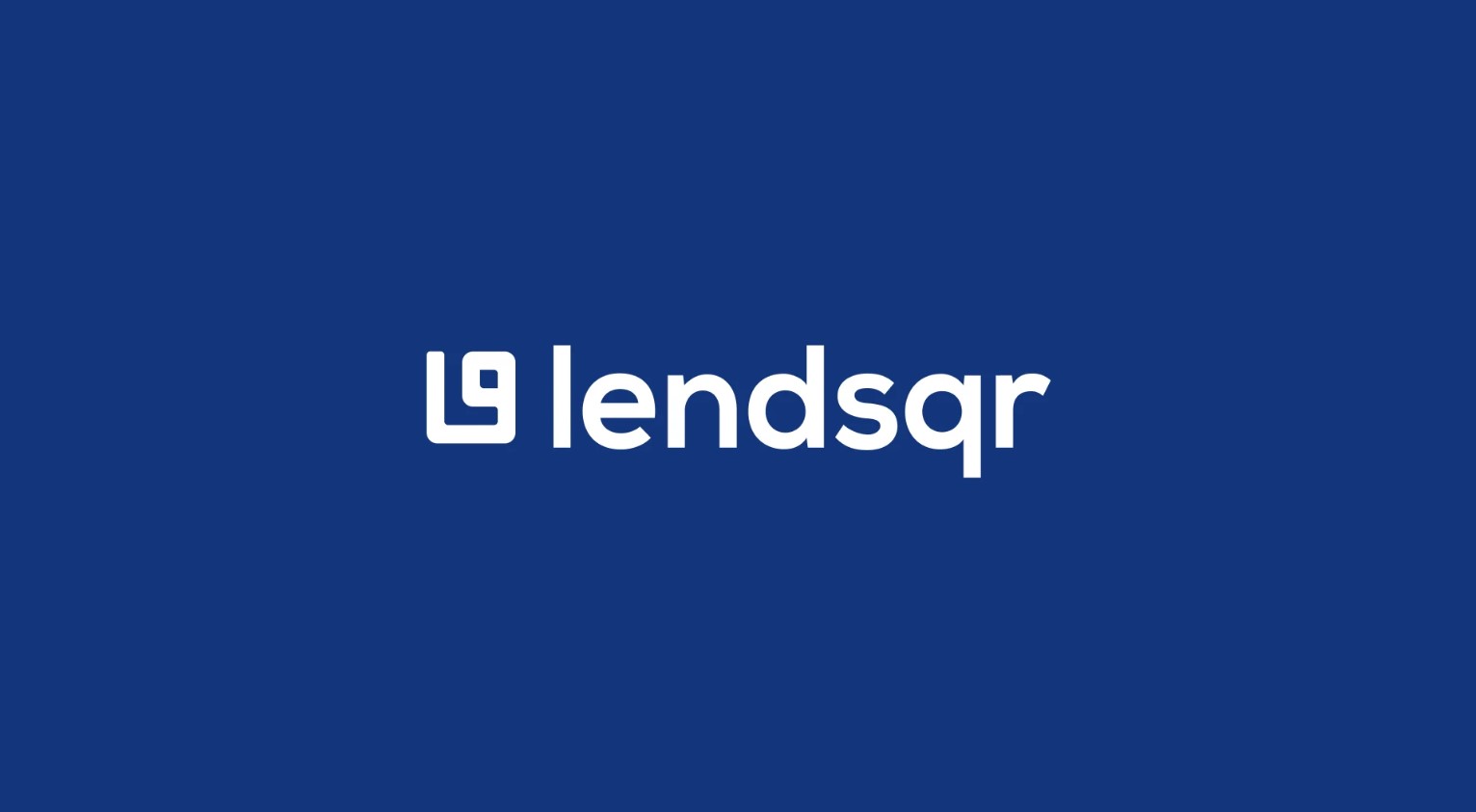
Chatbots are increasingly supplementing the efforts of human customer support staff. Health Maintenance Organisations (HMOs) now offer telemedicine services as a more convenient option for clients living in areas with limited access to primary healthcare centres.
While it may seem that AI has done nothing but impress companies by enhancing their operations in recent times, there have also been some troubling moments. In 2018, global e-commerce giant, Amazon, reversed its decision to adopt an AI-powered hiring system after it repeatedly exhibited bias against female applicants. Rather than assessing the merit of job applications based on parameters such as qualifications and experience, the system made decisions influenced by gender bias. More male candidates were shortlisted, while many qualified female applicants were simply dismissed.
During the training phase of Amazon’s AI model, it was exposed to job application patterns that reflected a higher number of male applicants than female ones. Consequently, the system developed a flawed assumption that men were more suitable for roles at Amazon.
While Amazon’s case highlighted gender bias, history has also shown that AI tools, if left unchecked, can exhibit racist tendencies. In 2016, a Microsoft chatbot named Tay was deactivated on Twitter after making several controversial comments about sensitive topics such as the Holocaust. Designed to learn from user tweets, Tay was soon bombarded with malicious content by users. It wasn’t long before the chatbot publicly denied that the Holocaust had ever occurred.
A significant amount of effort goes into preparing an AI model for a specific use case. Whether it is designed for telemedicine or loan applications, the AI tool must be trained using specific data to enable it to make informed decisions. The data used to train the model must be free from bias to prevent incidents like those previously mentioned.
Lendsqr’s proposed approach did not emerge in a vacuum; Africa’s lending system has been in need of a facelift for many years. Under the current structure, most people who submit loan applications without an existing credit history risk being denied outright, with little room for compromise.
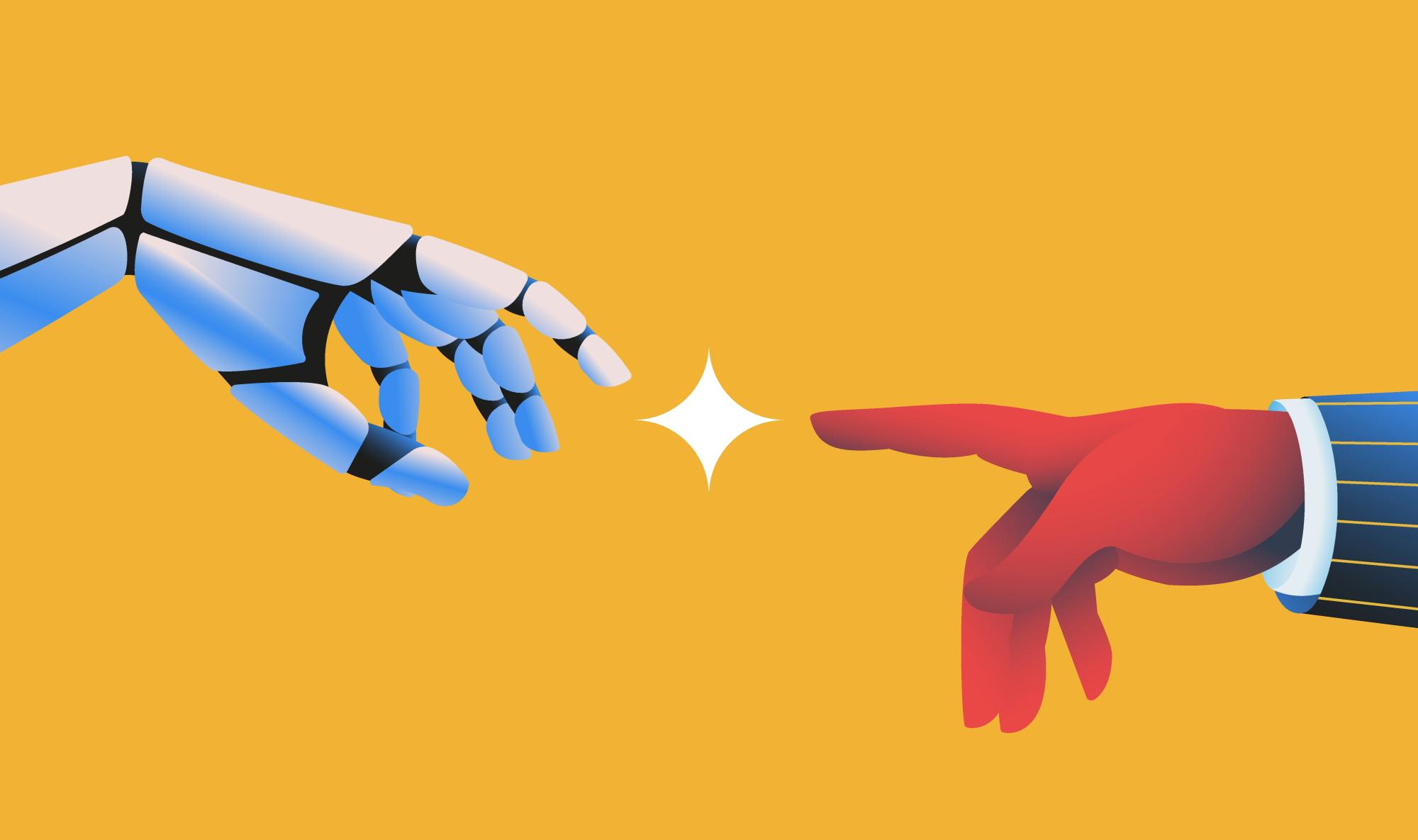
In Sub-Saharan Africa, approximately 80 million adults do not have bank accounts. As a result, they lack credit histories and are likely to be denied loans, regardless of their financial situation. By choosing not to judge applications solely on submitted documents and instead assessing applicants through voice or video chat, this AI model could provide access to loans for individuals who would previously have been excluded.
An AI-driven loan assessment system also makes sense, as it can help companies reduce costs—particularly those associated with salaries. Since the evaluation process would be handled by a machine that does not draw a salary, companies can redirect those funds towards other operational needs.
Although the prospect of automation is appealing, some ethical considerations must be highlighted. As seen in the cases of Tay and Amazon, the quality of training an AI model receives is crucial. Every effort must be made to eliminate bias during this stage. If one or more biases are allowed to persist in the training data, the tool may end up accepting or rejecting applicants based on factors such as ethnicity, financial background, age, or gender.
Therefore, companies like Lendsqr must tread carefully when developing an AI system for loan applications. They must ensure that no form of bias is introduced into the algorithms during training. This will help reduce the likelihood of applicants being denied for controversial reasons, such as age or income bracket.
Even if the idea of an AI-led loan officer sounds innovative, there remains a risk that applicants could lose the human connection typically found in interactions with a loan officer. An AI system may struggle to grasp certain contexts—such as sudden hardships or informal information—and could, as a result, exclude otherwise deserving applicants from consideration.
However, a human officer, guided by empathy, may be more willing to listen to an applicant’s explanation of informal income sources or how a sudden hardship has impacted their earning power. Therefore, retaining human staff to work alongside the AI tool will ensure that applicants can still connect on an emotional level—an attribute machines inherently lack.
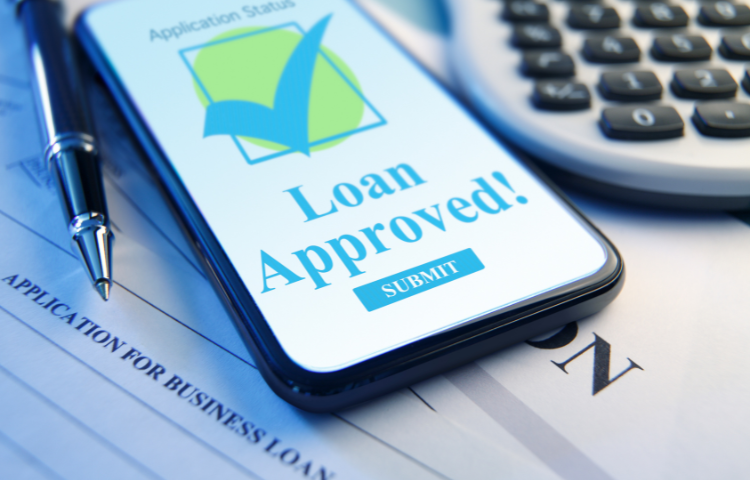
There is also the issue of data privacy. Conversing with an AI tool during a loan application process should, in principle, require companies to obtain consent before collecting voice or facial data. However, corporations are not widely known for adhering to such protocols, as seen in the cases of Meta and Google.
The absence of clear data privacy laws tailored to AI technologies may create uncertainty around the adoption of AI models for reviewing loan applications. African countries must step up efforts to develop modern data privacy legislation that not only protects citizens but also clearly outlines how applicants’ data can be used and the limits of such usage.
Africa’s lending system is long overdue for a transformation, and AI tools may well be the catalyst needed to revolutionise how loan applications are assessed. However, companies must pay close attention to the associated risks and ensure a cautious approach. For example, having a human worker supervise the AI system helps prevent unfair treatment of applicants. It also eases fears of AI replacing human jobs and instead promotes the idea of human–machine collaboration.
Michael Akuchie is a tech journalist with five years of experience covering cybersecurity, AI, automotive trends, and startups. He reads human-angle stories in his spare time. He’s on X (fka Twitter) as @Michael_Akuchie & michael_akuchie on Instagram.
Cover photo credit: Lehigh University